Section Computational Neuroimaging
The section for Computational Neuroimaging focuses on the development and application of artificial intelligence (AI) in medical imaging. This is one of the most exciting areas of research in medicine, since AI could help to improve the accuracy of the detection, diagnosis, and treatment of diseases. Our research at the interface of medicine and computer science has a strong translational character and is intended to transform clinical observations into testable research hypotheses and translate research findings – by using state-of-the-art technologies such as AI – into scientific advances.
The specific areas of research are centered around the application of AI in the field of neuroradiology, where we have previously developed methods for quantitative assessment of disease burden and evaluation of drug efficacy in patients with brain tumors and multiple sclerosis. Moreover, we have previously developed AI methods for high-throughput imaging biomarker discovery (radiomics) and demonstrated its future potential in various aspects of neuro-oncology for predictive modeling. By integrating our development into the most widely used informatics platform for imaging research (www.xnat.org) and through open-source deposition of our developments (www.neuroAI-HD.org) we pave the way for wide-spread application in the future. Ongoing and future research efforts are directed towards (a) large-scale validation and refinement of previous developments in controlled clinical settings, (b) identification of specific non-invasive surrogates for hallmark molecular alterations and subtypes in brain tumors, the “virtual biopsy” concept, to inform and refine molecularly guided treatment decisions, (c) to investigate the use of AI for image based predictive modeling in patients with stroke or multiple sclerosis, and (d) to develop explainable AI solutions which is an emerging subfield of AI seeking to understand how AI models generate their decisions.
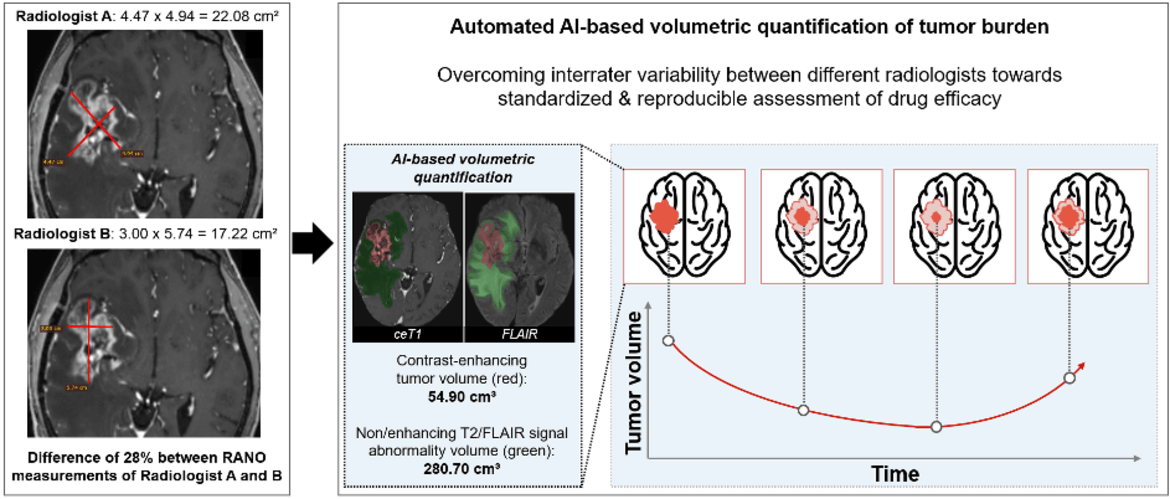
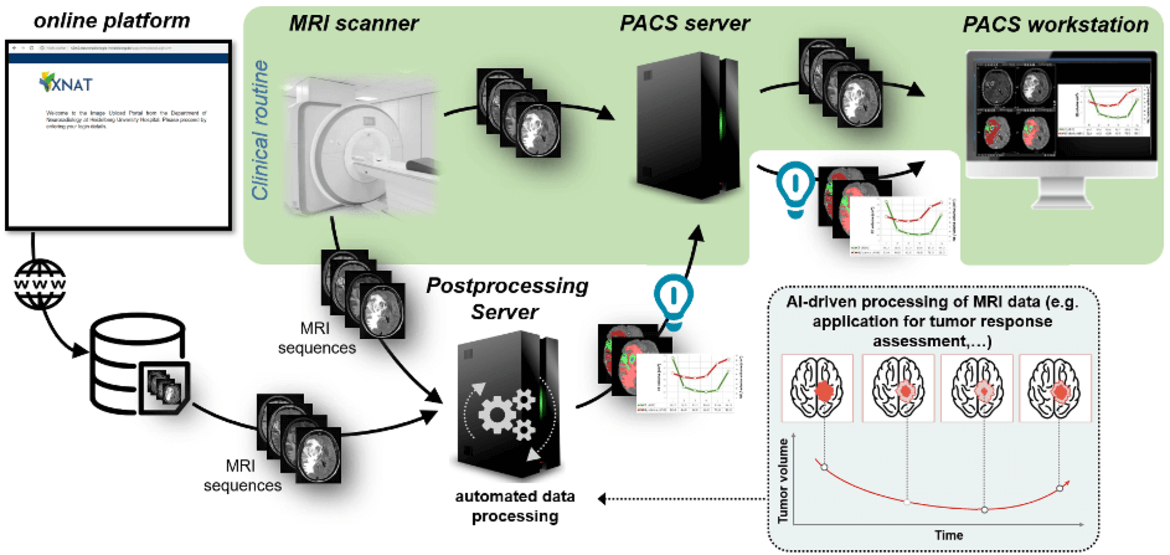
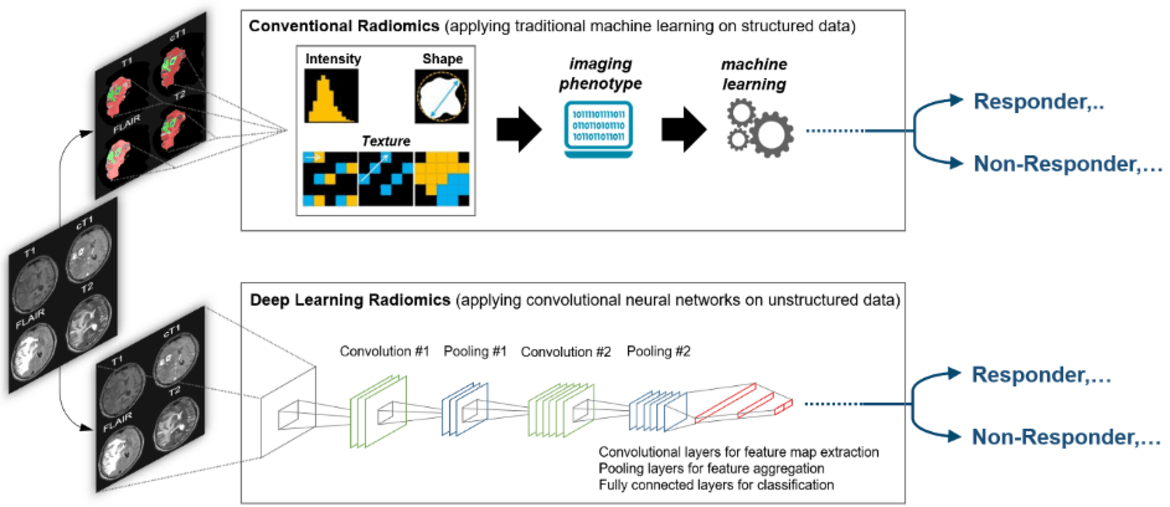
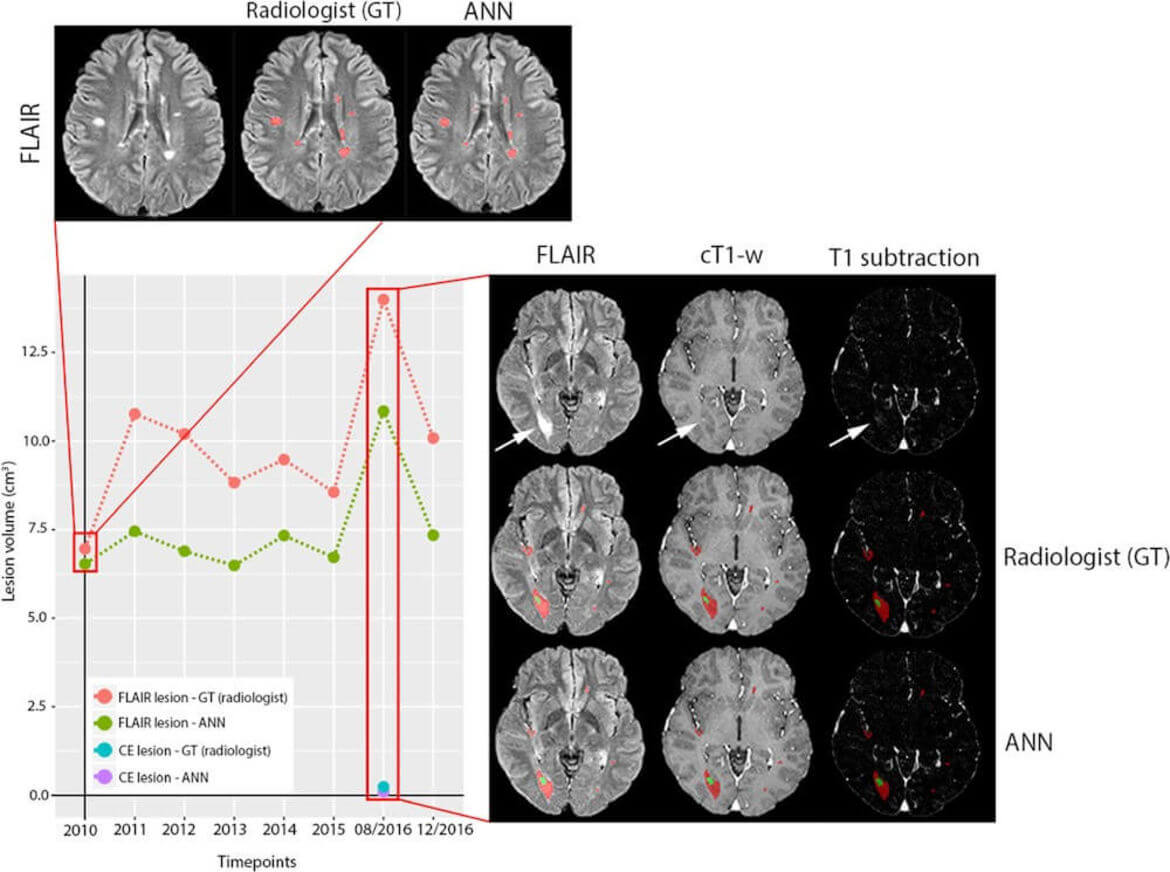
External Cooperations
- European Organization for Research and Treatment of Cancer (EORTC), Brussels
- German Cancer Research Center (DKFZ) (Department of Radiology & Medical Image Computing), Heidelberg
- Martinos Center for Biomedical Imaging & Massachusetts General Hospital (Department of Radiology) & Brigham and Women's Hospital (Dep. of Radiology), Boston, USA
- Cercare Medical, Aarhus, Denmark
Grants and Awards
Martha Foltyn:
- since 2020: Physician Scientist Fellowship from the Medical Faculty at Heidelberg University
Philipp Vollmuth (geb. Kickingereder):
- 2020: Research Prize from the Association for the Promotion of Neurological Sciences (NeuroWiss)
- 2020: Life Science Bridge Award of the Aventis Foundation (https://bridge.aventis-foundation.org/news/award-ceremony-2020/)
- 2019: Gerhard Domagk Prize for Cancer Research (awarded by the Foundation of Gerhard Domagk, Nobel Laureate in Physiology or Medicine, 1939)
- since 2019: Principal investigator in the Priority Program "Radiomics: Next Generation of Biomedical Imaging" funded by the Germany Research Foundation (DFG)
- since 2019: Principal investigator in the Collaborative Research Center "Understanding and Targeting Resistance in Glioblastoma" (SFB 1389) - www.unite-glioblastoma.de
- 2018-2020: Principal investigator within an academic / industrial partnership for developing novel AI-based decision support to improve the treatment of stroke patients funded by EUROSTARS (www.eurostars-eureka.eu)
- 2018: Semaan Family Foundation pilot grant (joint funding between Massachusetts General Hospital/Harvard Medical School and Heidelberg University Hospital)
- 2018: RADIOLOGY Editor's Recognition Award from the Radiological Society of North America (RSNA)
- 2018: Else-Kröner Memorial Fellowship from the Else Kröner-Fresenius Foundation
- 2016: Kurt-Decker-Award from the German Society of Neuroradiology (DGNR)
- 2015: Physician Scientist Fellowship from the Medical Faculty at Heidelberg University
- 2014: Marc-Dünzl-Award from the German Society of Neuroradiology (DGNR)
Ulf Neuberger:
- since 2020: Physician Scientist Fellowship from the Medical Faculty at Heidelberg University
Irada Pflüger:
- since 2020: Postdoc Fellowship through the Else Kröner Research College for Young Physicians
List of Publications
Selected Publications
- Schell M, Pflüger I, Brugnara G, Isensee F, Neuberger U, Foltyn M, Kessler T, Sahm F, Wick A, Nowosielski M, Heiland S, Weller M, Platten M, Maier-Hein KH, von Deimling A, van den Bent MJ, Gorlia T, Wick W, Bendszus M, Kickingereder P. Validation of diffusion MRI phenotypes for predicting response to bevacizumab in recurrent glioblastoma: post-hoc analysis of the EORTC-26101 trial. Neuro Oncol. 2020 May 11
- Brugnara G, Isensee F, Neuberger U, Bonekamp D, Petersen J, Diem R, Wildemann R, Heiland S, Wick W, Bendszus M, Maier-Hein KH, Kickingereder P. Automated volumetric assessment of multiple sclerosis disease burden and activity with artificial neural networks. Eur Radiol. 2020 Jan 3. [Epub ahead of print]
- Kickingereder P, Isensee F, Tursunova I, Petersen J, Neuberger U, Bonekamp D, Brugnara G, Schell M, Kessler T, Foltyn M, Harting I, Sahm F, Prager M, Nowosielski M, Wick A, Nolden M, Radbruch A, Debus J, Schlemmer HP, Heiland S, Platten M, von Deimling A, van den Bent MJ, Gorlia T, Wick W, Bendszus M, Maier-Hein KH. Automated quantitative tumor response assessment of MRI in neuro-oncology with artificial neural networks: a multicenter, retrospective study. Lancet Oncol. 2019
- Isensee F, Schell M, Pflueger I, Brugnara G, Bonekamp D, Neuberger U, Wick A, Schlemmer HP, Heiland S, Wick W, Bendszus M, Maier-Hein KH, Kickingereder P. Automated brain extraction of multi-sequence MRI using artificial neural networks. Hum Brain Mapp, 2019; 1-13.
- Kickingereder P, Neuberger U, Bonekamp D, Piechotta PL, Götz M, Wick A, Sill M, Kratz A, Shinohara RT, Jones DTW, Radbruch A, Muschelli J, Unterberg A, Debus J, Schlemmer HP, Herold-Mende C, Pfister S, Deimling AV, Wick W, Capper D, Maier-Hein KH, Bendszus M. Radiomic subtyping improves disease stratification beyond key molecular, clinical and standard imaging characteristics in patients with glioblastoma. Neuro Oncol. 2018 Oct 9;20(11):1517-1524.
- Tejada Neyra MA, Neuberger U, Reinhardt A, Brugnara G, Bonekamp D, Sill M, Wick A, Jones DTW, Radbruch A, Unterberg A, Debus J, Heiland S, Schlemmer HP, Herold-Mende C, Pfister S, von Deimling A, Wick W, Capper D, Bendszus M, Kickingereder P. Voxel-wise radiogenomic mapping of tumor location with key molecular alterations in patients with glioma. Neuro Oncol. 2018 Oct 9;20(11):1517-1524.
- Kickingereder P, Götz M, Muschelli J, Wick A, Neuberger U, Shinohara RT, Sill M, Nowosielski M, Schlemmer HP, Radbruch A, Wick W, Bendszus M, Maier-Hein KH, Bonekamp D. Large-scale Radiomic Profiling of Recurrent Glioblastoma Identifies an Imaging Predictor for Stratifying Anti-Angiogenic Treatment Response. Clin Cancer Res. 2016 Dec 1;22(23):5765-5771.
- Kickingereder P, Burth S, Wick A, Götz M, Eidel O, Schlemmer HP, Maier-Hein KH, Wick W, Bendszus M, Radbruch A, Bonekamp D. Radiomic Profiling of Glioblastoma: Identifying an Imaging Predictor of Patient Survival with Improved Performance over Established Clinical and Radiologic Risk Models. Radiology. 2016 Sep;280(3):880-9.
- Kickingereder P, Bonekamp D, Nowosielski M, Kratz A, Sill M, Burth S, Wick A, Eidel O, Schlemmer HP, Radbruch A, Debus J, Herold-Mende C, Unterberg A, Jones D, Pfister S, Wick W, von Deimling A, Bendszus M, Capper D. Radiogenomics of Glioblastoma: Machine Learning-based Classification of Molecular Characteristics by Using Multiparametric and Multiregional MR Imaging Features. Radiology. 2016 Dec;281(3):907-918.
- Kickingereder P, Sahm F, Radbruch A, Wick W, Heiland S, Deimling Av, Bendszus M, Wiestler B. IDH mutation status is associated with a distinct hypoxia/angiogenesis transcriptome signature which is non-invasively predictable with rCBV imaging in human glioma. Sci Rep. 2015 Nov 5;5:16238.
Downloads
Our key developments are available for download via https://github.com/NeuroAI-HD