Research Group Artificial Intelligence and Cognitive Robotics
About us
Welcome to the homepage of AICOR! As a research group within the division for minimally invasive and robot-assisted surgery we investigate methods of Artificial Intelligence and Cognitive Robotics (AICOR) to improve surgical treatment with a strong focus on clinical translation. We want to help surgeons to choose the best treatment option for the individual patient and perform the surgical intervention in the best possible way.
We believe that multidisciplinary collaboration is necessary to leverage the potential of digital technologies in surgery. Thus, our group brings together surgeons, medical students, computer scientists, roboticists, and designers to create novel solutions for clinical problems in surgery together with our cooperation partners from academia and industry. To optimize treatment decisions for surgical patients, we create decision support systems that recommend the best possible operative procedure or (neo)adjuvant therapy for surgical oncology patients or predict life threatening postoperative complications. To optimize the surgical intervention itself, we investigate cognitive surgical robots that co-operate with human surgeons to improve efficiency and safety in their operative workflow to perform the operation as good as possible.
Projects "Artificial Intelligence"
SurgOmics
Surgomics - Personalized prediction of life-threatening complications in surgery via machine learning on multimodal process data
The Surgomics project aims to predict life-threatening complications during and after oncological surgeries focusing on esophageal and pancreatic procedures. The project is a cooperation with the chair for translational surgical oncology at NCT Dresden and the department for visceral, thoracic and vascular surgery at Carl Gustav Carus University Hospital Dresden, as well as companies Karl Storz GmbH & Co KG and Phellow Seven GmbH.
At Heidelberg University Hospital the project involves our group within the surgical department and the division medical information systems within the hospital's IT-department.
Using artificial intelligence it is our vision to provide decision support and warning signals to the surgeon and therefore improve the patients´ prognosis. To reach this goal we will develop a holistic data model representing the surgical treatment path from the admission of the patient, over the operation room to the postoperative course ending with the patient’s discharge. Here, we will combine multimodal data about comorbidities and from preoperative imaging with intraoperative video data and medical device data for machine learning methods such as uncertainty-aware deep bayesian networks as well as graph neural networks. Integrating these features, we aim to realize a Cognitive Surgical Assistant (CoSA) that predicts postoperative complications before they occur and warns the surgeons at the bedside via a mobile app.
Principal Investigators Surgery Heidelberg:
- Prof. Beat Müller (PI)
- Dr. Martin Wagner (Deputy-PI)
Cooperation Partners:
- Prof. Stefanie Speidel, Dr. Sebastian Bodenstedt, Department for Translational Surgical Oncology, NCT Dresden (project coordinator)
- Prof. Jürgen Weitz, Prof. Marius Distler, Department for Visceral, Thoracic and Vascular Surgery, Carl Gustav Carus University Hospital Dresden
- Dr. Oliver Heinze, Division of Medical Information Systems, Heidelberg University Hospital
- Dr. Johannes Fallert, Dr. Lars Mündermann, Karl Storz GmbH & Co KG, Tuttlingen
- Nicolas Weiß, Maurice Fahn, Phellow Seven GmbH, Heidelberg
Funding:
Data Science driven Surgical Oncology
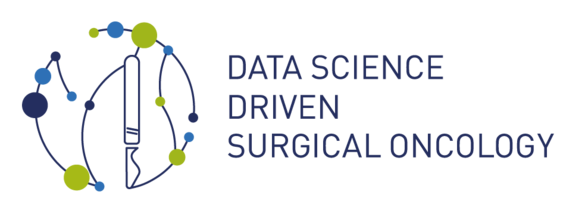
Data Science driven Surgical Oncology
In this project with computer scientists at the German Cancer Research Center (DKFZ) we aim to establish the field of surgical data science in the complex research and clinical environment of the National Center for Tumor Diseases (NCT Heidelberg) to enable personalized treatment decisions for patients with esophageal and liver cancer based on data-driven approaches.
Digitalization in medicine is leading to a continuously increasing volume of data. Over the course of treatment, many different types of data are collected. These include patient demographic data, laboratory values, radiological image data, reports from various disciplines in text form, high-resolution video recordings of laparoscopic operations, medical device data from the operating room and the intensive care unit and so on.
These different types of data are systematically recorded along the entire treatment path and create requirements for a novel surgical data science infrastructure that bridges Heidelberg University Hospital and DKFZ within the NCT. Based on this data and infrastructure, we aim to integrate data-based insights into clinically useful AI-applications presenting data in an understandable and trustworthy way to support surgical decision making.
Principal Investigators Surgery Heidelberg:
- Prof. Beat Müller (PI Surgery)
- Dr. Martin Wagner (Scientific Coordinator Medicine)
Cooperation Partners:
- Prof. Lena Maier-Hein (PI Data Science), Division of Computer-Assisted Medical Interventions, DKFZ Heidelberg
- Prof. Klaus Maier-Hein, Division of Medical Image Computing, DKFZ Heidelberg
- Prof. Annette Kopp-Schneider, Division of Biostatistics, DKFZ Heidelberg
- Dr. Oliver Heinze, Division of Medical Information Systems, Heidelberg University Hospital
Funding:
Additional Information:
Endoscopic Vision Challenges
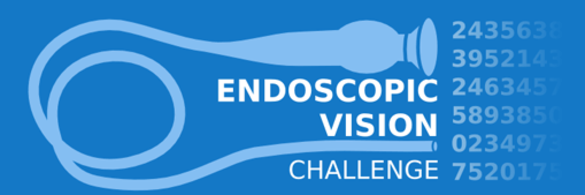
Endoscopic Vision Challenges
Benchmarking of machine learning algorithms is a prerequisite for clinical translation, because it allows to test, validate and compare algorithms in a scientific environment before they enter clinical applications. Since 2017 together with our research partners we have organized sub-challenges of the Endoscopic Vision Challenge held in conjunction with MICCAI in order to provide data scientists with open surgical datasets to benchmark their algorithms. Hereby, we aim not only to advance the field of surgical data science with open data, but also guide research into clinically meaningful directions by challenging research groups from all over the world with our datasets and computer vision tasks.
Cooperation Partners:
- Prof. Stefanie Speidel, Dr. Sebastian Bodenstedt, Department for Translational Surgical Oncology, NCT Dresden
- Prof. Lena Maier-Hein, Computer-Assisted Medical Interventions, DKFZ Heidelbeerg
- Prof. Franziska Mathis-Ullrich, Health Robotics and Automation Lab, KIT Karlsruhe
Additional Information:
Surgical Data Annotation
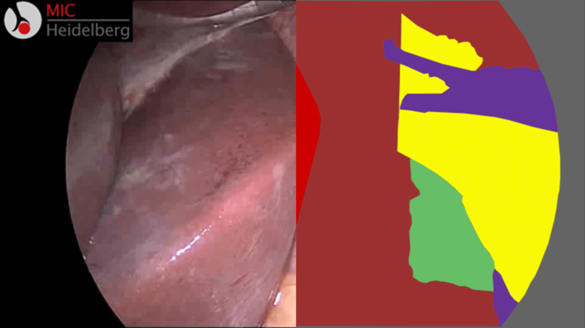
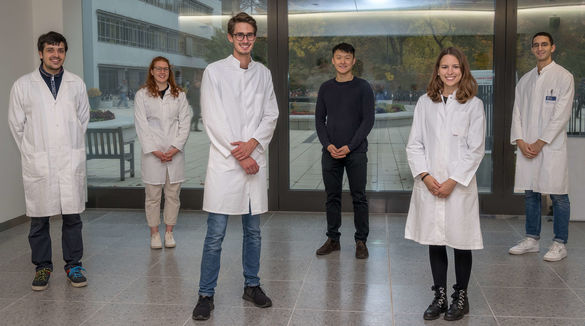
Surgical Data Annotation Team & Research on Artificial Intelligence for Surgical Data Annotation
Minimally invasive surgery creates a large quantity of raw, valuable image data such as laparoscopic videos. Effective use of this surgical data requires time-consuming pixelwise or framewise annotation by surgical experts. Because trained surgeons have limited time, the goal of our research in this project is to maximize the annotation quality and efficacy whilst minimizing the annotational effort through integrating active learning for data pre-processing and data selection.
In order to create high quality annotation, our Surgical Data Annotation Team (SDAT) is annotating surgical data, and the results are correlated via multi-rater-comparison. We also collect valuable metadata and iteratively evaluate the annotation process to maximize data quality. Our teams’ expertise covers the pixelwise semantic image segmentation of surgical images as well as the temporal, framewise annotation of surgical videos.
As a service to our data-hungry research partners we annotate surgical data for the use in supervised machine learning as a basis for context-aware surgical assistance, robot-assisted surgery and endoscopic vision challenges.
Cooperation partners:
Projects "Cognitive Robotics"
What is Koala?
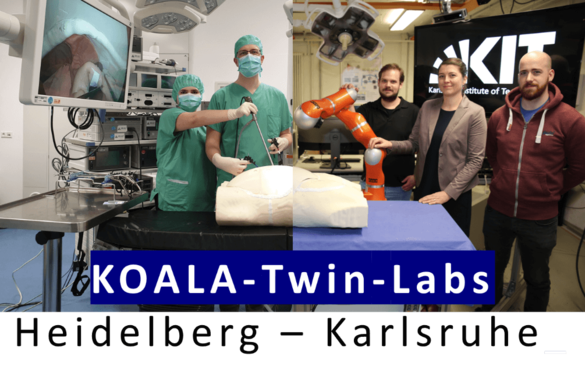
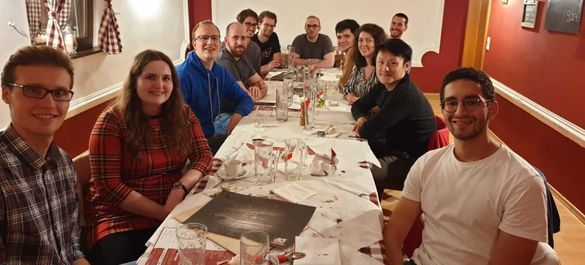
Together with Franziska Mathis-Ullrich and her team of the Health Robotics and Automation (HERA) Lab at Karlsruhe Institute of Technology (KIT) in 2019 we started a long-term endeavour of investigating methods, systems and applications of Cognitive Assistance in Laparoscopy (Koala) in order to automate laparoscopic cholecystectomy by 2050 and along this way translate applications of cognitive robots with shared autonomy into surgical reality. Since then, we started a number of projects for various sub-aspects of this topic.
Cooperation partners:
- Prof. Franziska Mathis-Ullrich, Health Robotics and Automation Lab, KIT Karlsruhe
- Prof. Stefanie Speidel, Dr. Sebastian Bodenstedt, Department for Translational Surgical Oncology, NCT Dresden
- Prof. Ralf Mikut, Automated Image and Data Analysis, KIT Karlsruhe
- Prof. Ali Sunyaev, Critical Information Infrastructures Research Group, KIT Karlsruhe
- Dr. Wolfgang Meier, AMS – Gesellschaft für Automatisierungs- und Meß-Systemtechnik GmbH, Karlsruhe
- Dr. Darko Katic, ArtiMinds Robotics GmbH, Karlsruhe
Koala-Grasp
Koala-Grasp - A learning robotic assistance system for surgical grasping- und holding tasks
Surgeons rely on surgical assistants for most daily procedures. The lack of surgical assistants is therefore a structural problem in surgical care, especially in remote areas. Koala-Grasp addresses this problem with the innovative use of robotic systems to relieve surgeons of their daily workload while providing the best patient care.
Thus, the aim of the project is to develop a robotic assistance system which allows gripping and holding tasks in minimally invasive soft tissue surgery to be learned and performed autonomously in a force-adapted and context-sensitive manner. In the beginning we take laparoscopic gallbladder removal as an example. To achieve this goal, a mechatronic interface will be developed to locally collect sensor data from laparoscopic instruments during robot-assisted surgery, based on which the robotic assistant will learn the grasping, as well as trajectory and manipulation strategies in order to support the surgeon during the operation in a context-aware manner. Through a differentiated preclinical validation and the answering of ethical, legal and social questions of (partially) autonomous robotic assistance systems in surgery, the foundations for a clinical translation are to be established.
Principal Investigators Surgery:
- Prof. Beat Müller (PI)
- Dr. Martin Wagner (Deputy-PI)
Cooperation partners:
- Dr. Wolfgang Meier (Project Coordinator), AMS – Gesellschaft für Automatisierungs- und Meß-Systemtechnik GmbH, Karlsruhe
- Prof. Franziska Mathis-Ullrich, Health Robotics and Automation Lab, KIT Karlsruhe
- Dr. Darko Katic, ArtiMinds Robotics GmbH, Karlsruhe
Funding:
Koala-MARS
Koala-MARS - Cooperative multi-agent reinforcement learning for next-generation cognitive robotics in laparoscopic surgery
Laparoscopic surgery is a team effort. A surgeon and her assistant(s) collaborate to solve a shared task working individually and as a team for a successful surgical outcome. However, our society faces an increasing shortage of skilled surgeons and assistants, especially in rural areas. This shortage may be resolved by providing cognitive surgical robots that automate certain tasks. Our project addresses the highly interdependent behavior of surgeon and assistant(s) as a multi-agent robotic surgery (MARS) system problem of human and artificial agents using methods of cooperative multi-agent reinforcement learning (cMARL). In contrast to previous work, this project aims to train multiple, decentralized artificial agents that cooperatively solve a shared, robot-assisted laparoscopic task.
Cooperation partners:
- Prof. Franziska Mathis-Ullrich, Health Robotics and Automation Lab, KIT Karlsruhe
- Prof. Ralf Mikut, Automated Image and Data Analysis, KIT Karlsruhe
Funding:
Koala-WORLD
Koala-WORLD - Learning a World Model of Surgical Oncology for Cognitive Assistance Systems in Laparoscopic Surgery
Minimally-invasive surgery is increasingly carried out by telemanipulation of robotic actuators by the surgeon to reduce physical strain and increase dexterity by means of tremor filtration and image magnification. This opens the door to partial or even full automation of simple or repetitive surgical subtasks, with the promise of reducing mental workload for the surgeon or even speeding up the operation. State-of-the-art reinforcement learning (RL) algorithms have been shown to learn complex behaviours from interaction with an environment while maximizing a reward signal. However, they have low sample efficiency, and must be retrained from scratch for each new task and environment. A surgical environment is particularly destructible and soft by nature, effectively precluding training an agent for robotic surgery in a real environment. We aim to overcome both limitations (low sample efficiency and difficult transferability) using the method of world models, and successfully transfer a RL policy trained to perform simple surgical tasks in simulation, into real world surgical applications. Model-based RL exhibits significantly higher sample efficiency than other methods as an agent can exploit its understanding of the environment dynamics to plan ahead. World models, a recent class of methods, extend this by learning and planning in a latent space rather than relying on raw pixel observations, allowing for efficient “imagining” of trajectories many time steps into the future. Because the model is learned not just simply end-to-end, certain elements of the policy can be transferred to the real world with minimal fine-tuning. Our goal is to extend world models to the unique problem of robotic surgery, and develop new methods in transfer learning to bring these benefits into the real world. The proposed project will support our vision of surgery & intervention 4.0 where a single surgical team may simultaneously supervise several remote surgeries, with all but the most critical maneuvers being carried out autonomously by cognitive surgical robots.
Cooperation partners:
- Prof. Franziska Mathis-Ullrich, Health Robotics and Automation Lab, KIT Karlsruhe
- Prof. Ralf Mikut, Automated Image and Data Analysis, KIT Karlsruhe
Funding:
Koala-GameIT
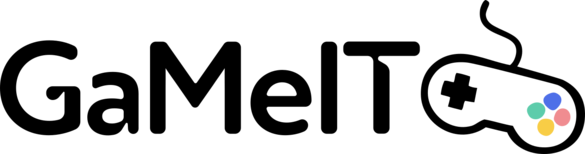
Koala-GameIT - Data-driven Gamification to Improve Quality in Medical Image Annotation Tasks
Cognitive surgical assistance systems, such as surgical robots require image-based scene understanding to perceive the surgery context, comprehend the surgery procedure, and eventually generate safe trajectories to assist during the surgery. To achieve such scene understanding, recognition and semantic segmentation of different surgery aspects (e.g., shown organs, used surgical tools, different surgery stages) are necessary pre-conditions. Since the development of such robotic assistance systems in surgery requires a lot of annotated surgical data, we are facing the problem that there is an enormous amount of raw data needing to be processed by surgical experts. Meanwhile, this task of annotating itself can be a tedious and time-consuming experience.
Therefore, we attempt to explore how we can improve the overall annotation process, making it more pleasant, time-efficient, motivating and hopefully entertaining.
In particular, we design, implement, and evaluate a data driven machine-learning-based gamification concept to foster annotators engagement and, thereby, ensure high quality data labeling. By drawing on machine learning methods, the gamification concept is able to adapt to individual user preferences and overcome the weaknesses of one-size-fits-all gamification approaches.
Cooperation partners:
- Prof. Ali Sunyaev, Critical Information Infrastructures Research Group, KIT Karlsruhe
- Prof. Franziska Mathis-Ullrich, Health Robotics and Automation Lab, KIT Karlsruhe
Funding:
Projects Miscellaneous
CatHERA
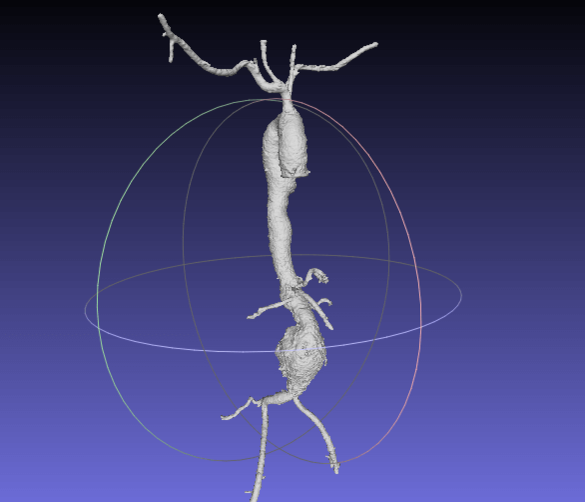
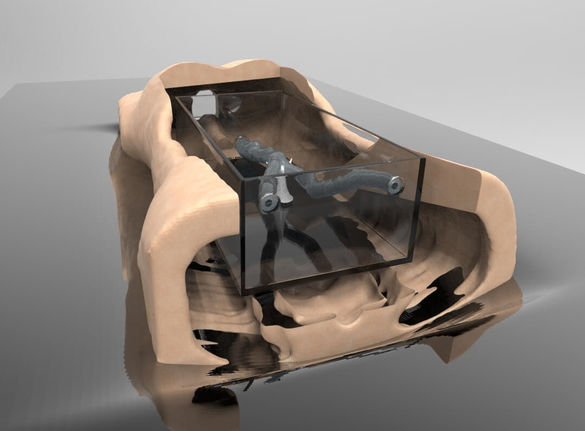
CatHERA - a reconfigurable simulation environment to optimize flexible instruments für medical applications
The CatHERA project aims to develop a reconfigurable simulation environment for intervention using flexible instruments. With this simulator, the project aims at two goals. On one hand we want to develop a medical test bed ("medical phantom") that simulates the geometries and material properties of several tubular human organs (e.g., blood vessels, lungs,) and allows to easily and reproducibly evaluate and iteratively optimize different designs and coatings of flexible instruments with respect to positioning accuracy and interaction forces on the tissue. On the other hand, this reconfigurable platform offers the possibility of a demonstrator where both training medical students and residents in vascular surgery can train medical application of flexible instruments in different organs or the interested public (e.g. patients, pupils) can experience vascular interventions in a safe environment.
Cooperation partners:
- Prof. Franziska Mathis-Ullrich, Health Robotics and Automation Lab, KIT Karlsruhe
- Prof. Dittmar Böckler, Dr. Katrin Meisenbacher, Department of Vascular and Endovascular Surgery, Heidelberg University Hospital
Funding:
MIC-Channel
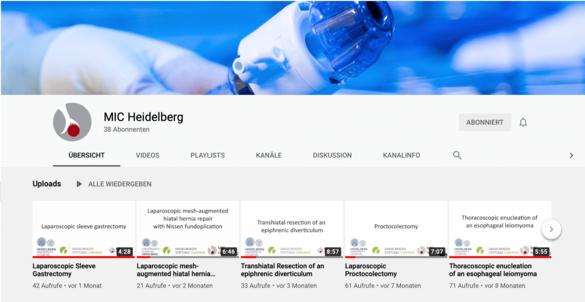
Youtube channel of the Section for Minimally Invasive and Robot-assisted Surgery (MIC)
MIC stands for “minimally invasive surgery“ and the MIC Channel is a Youtube channel created by our research group within the section for minimally invasive and robot-assisted surgery at Heidelberg University Hospital. It presents educational videos of several laparoscopic surgical procedures. Our content is made for surgeons, other physicians, people working in medicine, medical students and also people who are interested in general.
We have developed a quality management process for our videos that integrates teaching the next generation of future surgeons. Within our MIC channel medical students get the opportunity to edit a surgical video and within that process also improve their surgical and anatomical knowledge. The videos therefore present a perfect combination of learning about a surgical procedure while editing the video and receiving expert feedback from experienced surgeons that review the videos before publication.
Funding:
Additional Information:
REDCap
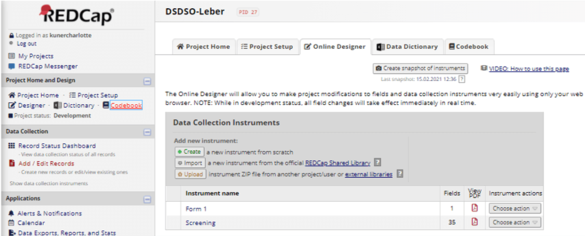
REDCap as a clinical database system for the study centre of the german surgical society (SDGC)
We are establishing the research electronic data capture (REDCap) system for the department of surgery under the roof of the study centre of the german surgical society (SDGC). Based on the infrastructure provided by the division of medical information systems, we ensure accordance to data privacy regulations and help surgeons to capture their data not in Excel, but an easy to handle, yet powerful database system.
Cooperation partners:
- Prof. Pascal Probst, Department of General, Visceral and Transplantation Surgery, Heidelberg University Hospital
- Dr. Oliver Heinze, Division of Medical Information Systems, Heidelberg University Hospital
Additional Information:
Team
Surgeon Scientist
PhD Candidates
-
Balazs Gyenes, M.Sc.
Robotics, Machine Learning. PhD-student associated with AICOR. Balazs' main affiliation is the HERA lab @ KIT.
Focus
Learning a World Model of Surgical Oncology for Cognitive Assistance Systems in Laparoscopic Surgery.
-
Paul Maria Scheikl, M.Sc.
Robotics, Machine Learning, Mechatronics. PhD-student associated with AICOR. Paul's main affiliation is the HERA lab @ KIT.
Focus
Cooperative multi-agent reinforcement learning for next-generation cognitive robotics in laparoscopic surgery.
-
Simon Warsinsky, M.Sc.
Gamification and Surgical Data Annotation. PhD student associated with AICOR. Simon’s main affiliation is the cii lab @ KIT.
Focus
Data-driven Gamification to Improve Quality in Medical Image Annotation Tasks (GaMeIT).
Medical doctoral candidates
-
Johanna Brandenburg
Intraoperative decision support, surgical workflow modeling, systematic reviews.
Focus
Process modeling and analysis for a context sensitive decision support system in oncological visceral surgery.
-
Jonathan Chen
Data annotation, Machine learning and data science using R and Python
Focus
Conception and validation of an intelligent software as part of a quality-controlled process for surgical image annotation.
-
Michael Haselbeck-Köbler
Systematic reviews, machine learning in surgical oncology
Focus
Development of a data-driven decision support system for oncological liver surgery.
-
Anna Kisilenko
Surgical workflow and skill annotation, cognitive robotics research
Focus
Development and evaluation of methods for training cognitive surgical robots on the example of laparoscopic cholecystectomy
-
Duc Tran
Surgical data annotation, REDCap
Focus
Development and evaluation of artificial intelligence methods for OR process management in the context-aware operating room.
-
Rayan Younis
Data annotation, surgical workflow modeling
Focus
Modeling surgical activities for cognitive robots.
Student assistants
-
Tornike Davitashvili
Surgical & radiological data annotation, Digital 3D-models of organs, Video editing
Focus
Surgical Robotics Research
-
Philipp Alexander Petrynowski
Surgical data annotation
-
Vincent Vanat
Alumni
- Charlotte Kuner (Student assistant)
- Frederic Myers, B.Sc. (Bachelor student interaction design)
- David Markovic Lubtsky, B.Sc. (Student assistant)
- Benjamin Müller (Student assistant)
- Marie Raddatz (Student assistant)
- Manuela Capek (Student assistant)
- Hannes Maurer, B.Sc. (Bachelor student interaction design)
- Sven Hornung, B.Sc. (Bachelor student interaction design)
- Mareike De Groot (Student assistant)
- Lukas Raedeker (Student assistant)
- Lena-Marie Ternes (Medical doctoral student)
- Patrick Mietkowski (Medical doctoral student)
- Rudolf Rempel (Medical doctoral student)
- Dr. med. Benjamin Meyer (Medical doctoral student)
Publications
Highlights
Defining the research field of Surgical Data Science as a partner in an international Consortium
Maier-Hein L, Vedula SS, Speidel S, Navab N, Kikinis R, Park A, Eisenmann M, Feussner H, Forestier G, Giannarou S, Hashizume M, Katic D, Kenngott H, Kranzfelder M, Malpani A, März K, Neumuth T, Padoy N, Pugh C, Schoch N, Stoyanov D, Taylor R, Wagner M, Hager GD, Jannin P
Surgical data science for next-generation interventions.
In: Nature Biomedical Engineering 2017
First annotated data set from the sensor operating room as a benchmark for Surgical Data Science
Maier-Hein L*, Wagner M*, Ross T, Reinke A, Bodenstedt S, Full PM, Hempe H, Mindroc-Filimon D, Scholz P, Tran TN, Bruno P, Kisilenko A, Müller B, Davitashvili T, Capek M, Tizabi M, Eisenmann M, Adler TJ, Gröhl J, Schellenberg M, Seidlitz S, Emmy Lai TY, Pekdemir B, Roethlingshoefer V, Both F, Bittel S, Mengler M, Mündermann L, Apitz M, Kopp-Schneider A, Speidel S, Kenngott HG†, Müller-Stich BP†
Heidelberg Colorectal Data Set for Surgical Data Science in the Sensor Operating Room.
In: Scientific Data 2021, *† - authors contributed equally
Comparative validation of machine learning algorithms for instrument segmentation in laparoscopy
Roß T*, Reinke A*, Full PM, Wagner M, Kenngott H, Apitz M, Hempe H, Mindroc-Filimon D, Scholz P, Tran TN, Bruno P, Arbeláez P, Bian G-B, Bodenstedt S, Lindström Bolmgren J, Bravo-Sánchez L, Chen H-B, González C, Guo D, Halvorsen P, Heng P-A, Hosgor E, Hou Z-G, Isensee F, Jha D, Jiang T, Jin Y, Kirtac K, Kletz S, Leger S, Li Z, Maier-Hein KH, Ni Z-L, Riegler MA, Schoeffman K, Shi R, Speidel S, Stenzel M, Twick I, Wang G, Wang J, Wang L, Wang L, Zhang Y, Zhou Y-J, Zhu L, Wiesenfarth M, Kopp-Schneider A, Müller-Stich BP, Maier-Hein L
Comparative validation of multi-instance instrument segmentation in endoscopy: Results of the ROBUST-MIS 2019 challenge.
In: Medical Image Analysis 2020, * - authors contributed equally
Translational study of a NOVEL 3D bowel measurement system
Wagner M, Mayer BFB, Bodenstedt S, Stemmer K, Fereydooni A, Speidel S, Dillmann R, Nickel F, Fischer L, Kenngott HG.
Computer-assisted 3D bowel length measurement for quantitative laparoscopy.
In: Surgical Endoscopy 2018
First use of the hybrid-OR in liver cancer surgery
Kenngott HG, Wagner M, Gondan M, Nickel F, Nolden M, Fetzer A, Weitz J, Fischer L, Speidel S, Meinzer HP, Böckler D, Büchler MW, Müller-Stich BP (2014).
Real-time image guidance in laparoscopic liver surgery: first clinical experience with a guidance system based on intraoperative CT imaging.
In: Surgical Endoscopy 2014